Sommaire
Hate messages and social media: The challenges of moderation
Trigger Warning: This article includes examples of racist and homophobic language.

The issue of online moderation is becoming an increasingly complex and significant societal debate. Elon Musk’s purchase of Twitter has further highlighted this thorny problem. However, moderating such content remains extremely difficult, especially when dealing with various sensitive issues. To address this, Saper Vedere conducted a study focusing on hateful keywords targeting a range of minorities. Out of a dataset of 140,161 tweets, after applying different filters (see methodology), 10,861 unique tweets were identified.
The study revealed important findings:
Far-right groups and the football world are the most active in deploying hateful language. Football fans have developed a homophobic rhetoric in their commentary on football news, often using terms such as “f****t” and other slurs to refer to players, opponents, referees, etc.
It appears that societal factors (specific events, political targets, far-right affiliation, or football culture) have a greater impact on the production of hateful content than the digital medium as a facilitator.
Most hate messages are comments with little visibility or virality. Over the span of a year, we identified only 2,099 tweets that were clearly insults and should have been moderated. 67% of these insults were replies to other tweets. When analyzing these replies, we discovered that they often targeted specific individuals (Mbappé, Macron, Bilal Hassani, etc.). Insults are not widely retweeted, unlike testimonies that include insults.
Automated language processing is an ineffective method for identifying hate speech due to semantic issues (e.g., “fly swatter”), irony, and the importance of context in qualifying content. Additionally, sentiment analysis algorithms on insults identified only 11% of negative content and even mistakenly labeled 4% as positive content. Manual moderation remains a highly effective option, given that only 2,099 insults were identified over a year, averaging just 6 hateful tweets per day. This is entirely manageable by content moderators. These moderators can be assisted by statistical probabilities since we identified several metadata correlations with hate speech:
•Clustering algorithms based on relationships between individuals: Far-right communities are responsible for the majority of insults.
Tweet format: 67% of hateful tweets are replies.
Use of pseudonyms: 94% of hateful tweets are posted under pseudonyms.
Gender: Excluding pseudonyms, 73% of insults come from individuals identified as male.
Events where minorities are in the media spotlight: Spikes in hate tweets occur around LGBT-related events or events perceived as LGBT by those issuing insults (e.g., Eurovision).
5 types of hate speech on Twitter
We identified five categories of hate speech targeting minorities:
Insults
Hateful content attacking a person or group based on attributes such as race, ethnicity, religion, gender, or sexual orientation. This content is clearly problematic.
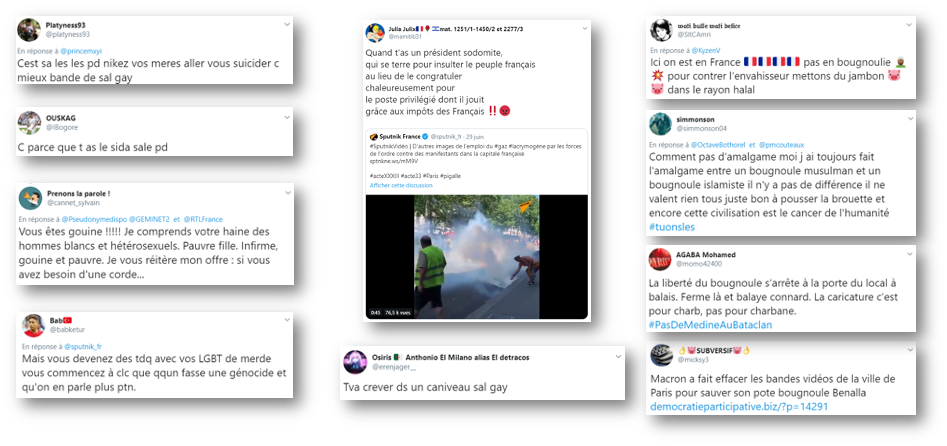
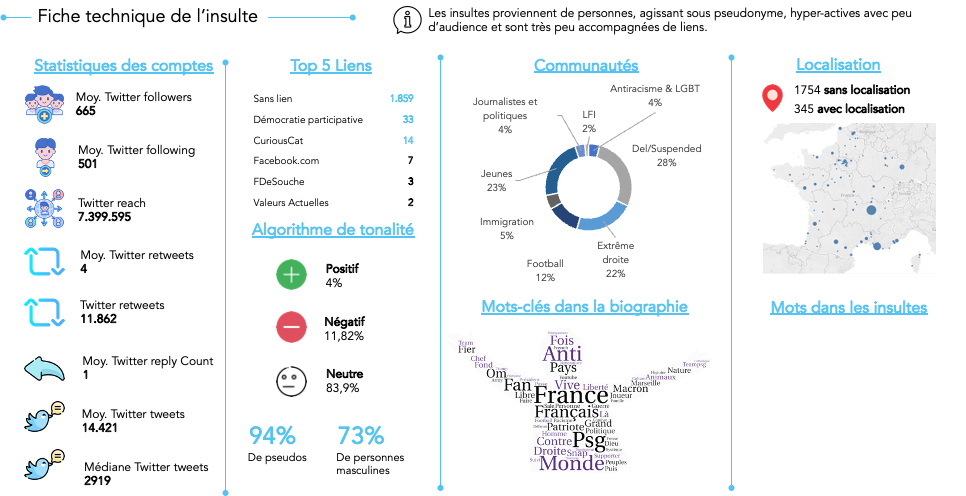
The two most targeted groups for insults are Arabs and homosexuals, despite the fact that mentions of homophobic insults were only analyzed over a three-month period rather than a full year.
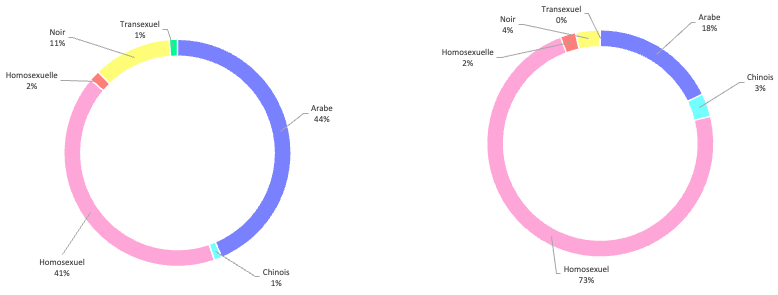
The insult “sale arabe” is the most frequently used slur, followed by homophobic insults and slurs targeting Black individuals.
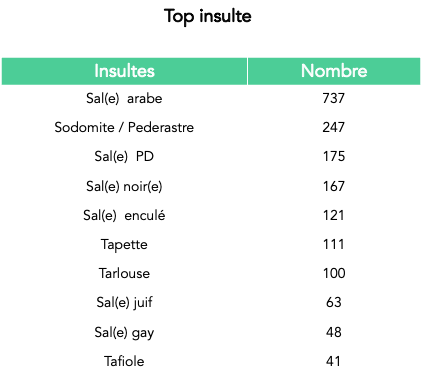
Denominative
Hateful language relating to race, ethnicity, religion, gender, or sexual orientation, but used without hateful intent. This could involve self-qualification (someone using insulting language to describe themselves or their behavior), negatively labeling a person, organization, or event (“What a ft that guy is”), or friendly, humorous interactions between acquaintances (“Big ft” to a gay friend; “Dirty Arab” to a friend of Arab origin; “Big Black guy” to a Black friend), as well as common expressions (“Don’t be a f****t,” “You’re playing like a sissy”).
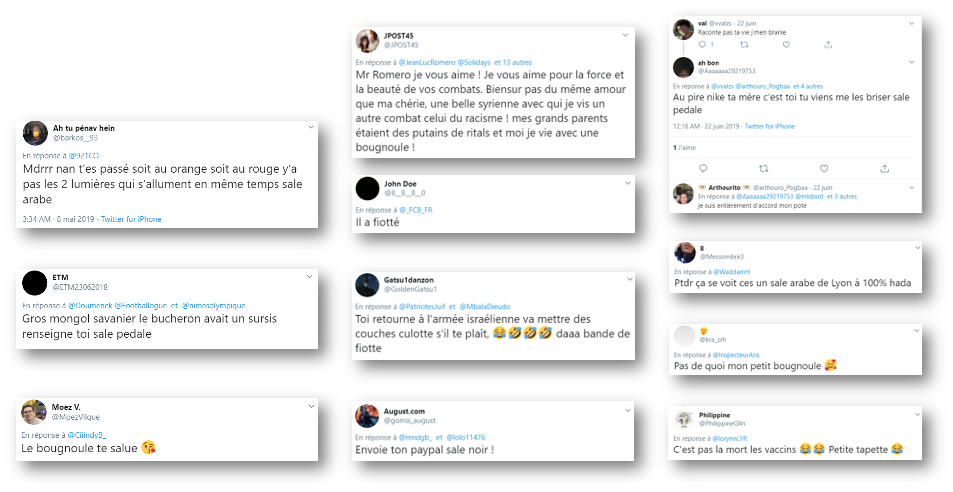
Declarative
Content reporting a hateful attack based on race, ethnicity, religion, gender, or sexual orientation. This could include situations from the victim’s childhood (insults, bullying, aggression from classmates, teachers’ failure to react), present-day situations (public insults, harassment, latent homophobia during family meals, racist or homophobic comments from police officers), or reports of incidents not personally experienced.
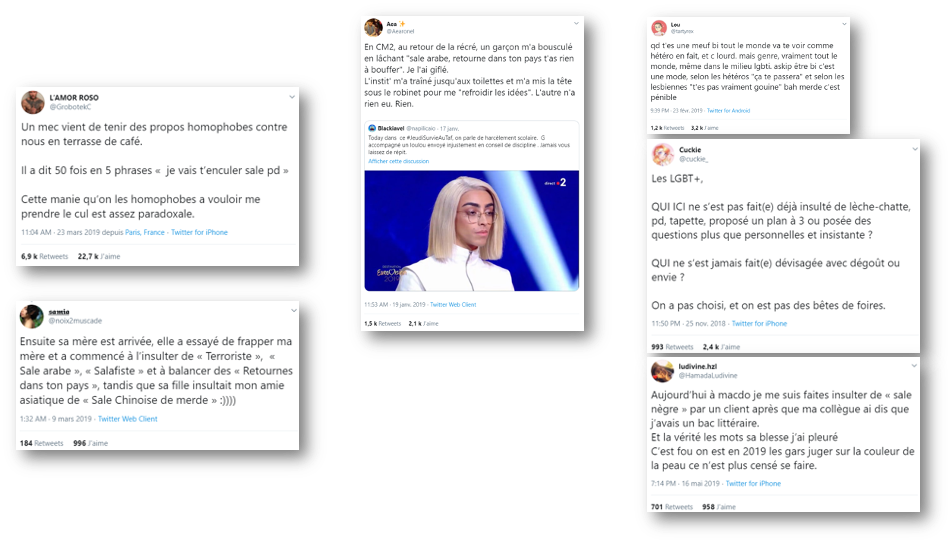
Irony
Hateful language related to race, ethnicity, religion, gender, or sexual orientation, but used in an ironic context. Irony is often employed with insults targeting specific communities (ethnicity, sexual orientation, etc.). This can be either in a defensive stance (responding to hate speech by ironically repeating the insulting arguments) or in a humorous context (using insults in a joke or by a member of the targeted community to mock a declaration or situation).
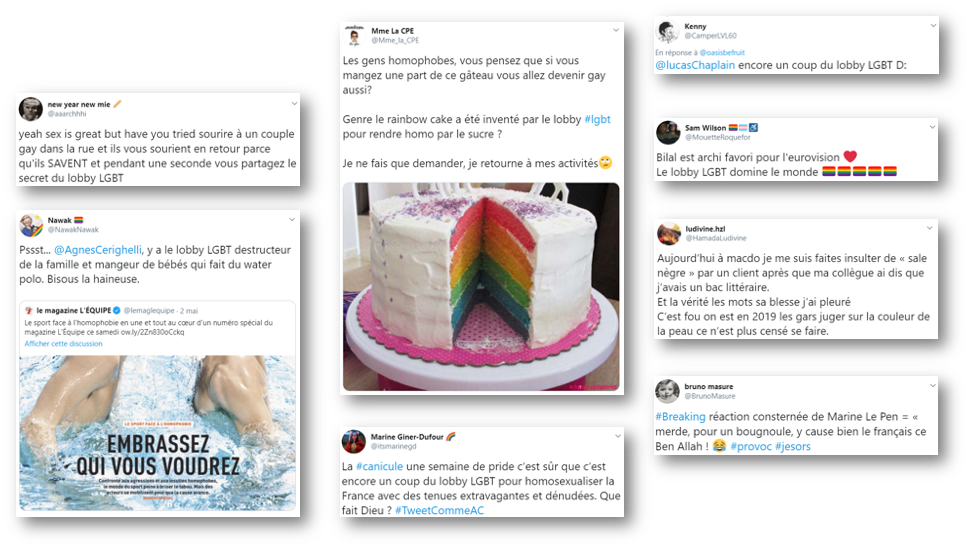
Tendentious Lobbying
Behind the denominative use of “lobbying” lies a tendentious mindset. We explore this in our study. The case of the "LGBT lobby" is particularly interesting as the narratives clearly lean towards conspiracy theories.
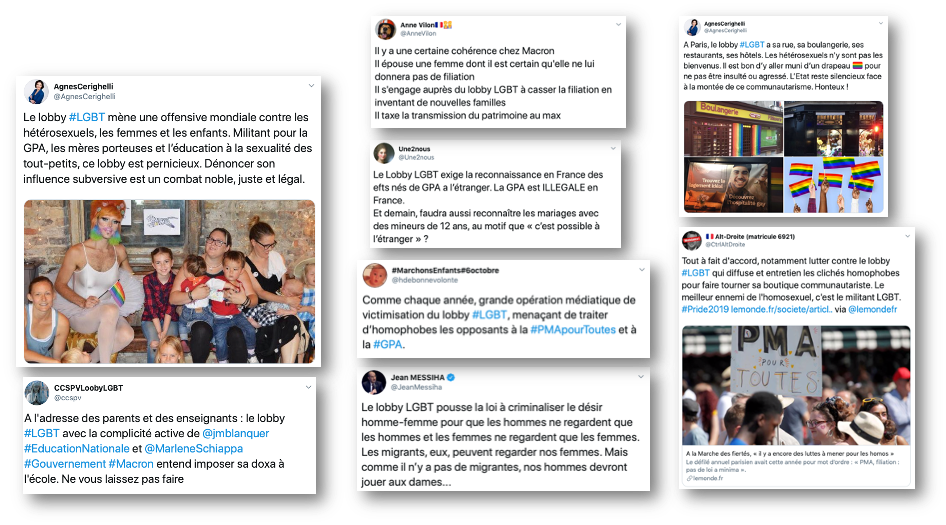
The same logic applies to the "Jewish lobby," where conspiracy theories are similarly at play.
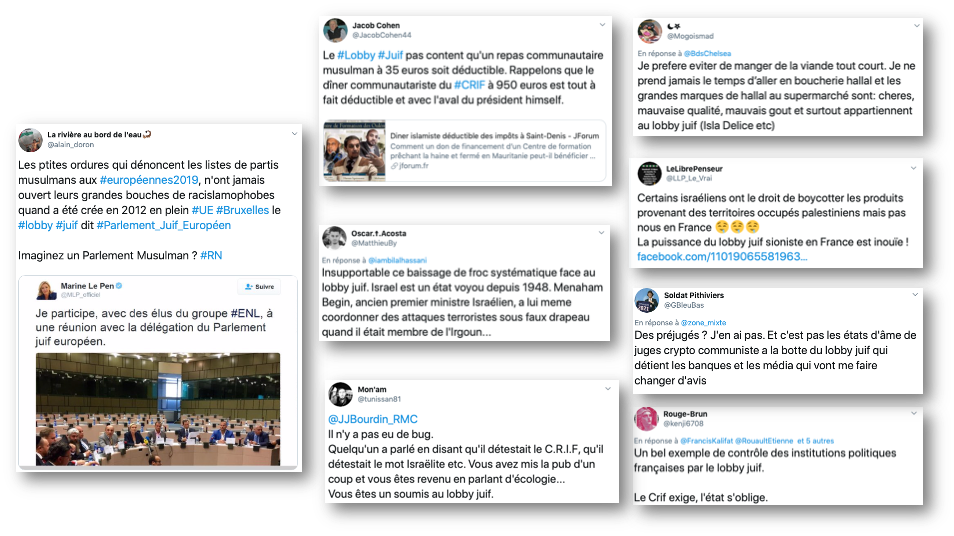
4 communities involved
In terms of actors, four main communities are involved: nationalists, public life, diversity, and sports:
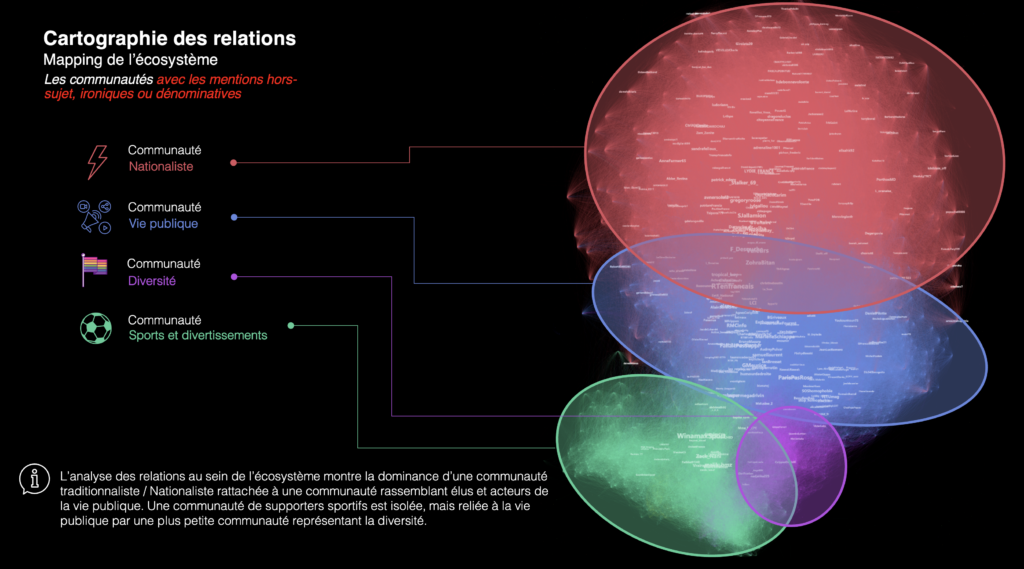
By zooming in on those who actually issue insults, we can isolate a community around PSG and a nationalist community:
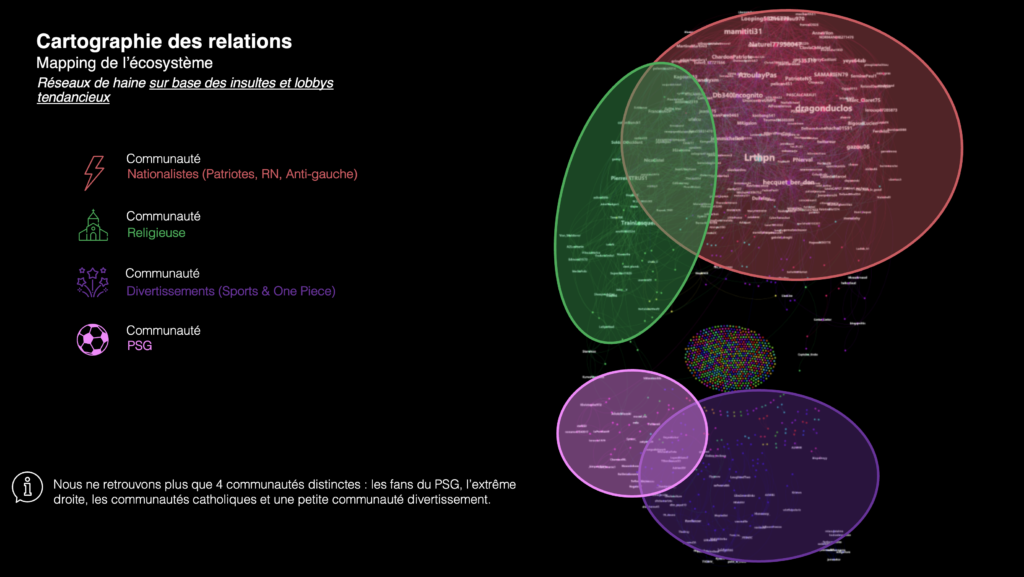
The far-right is indeed the most active community in this sphere:
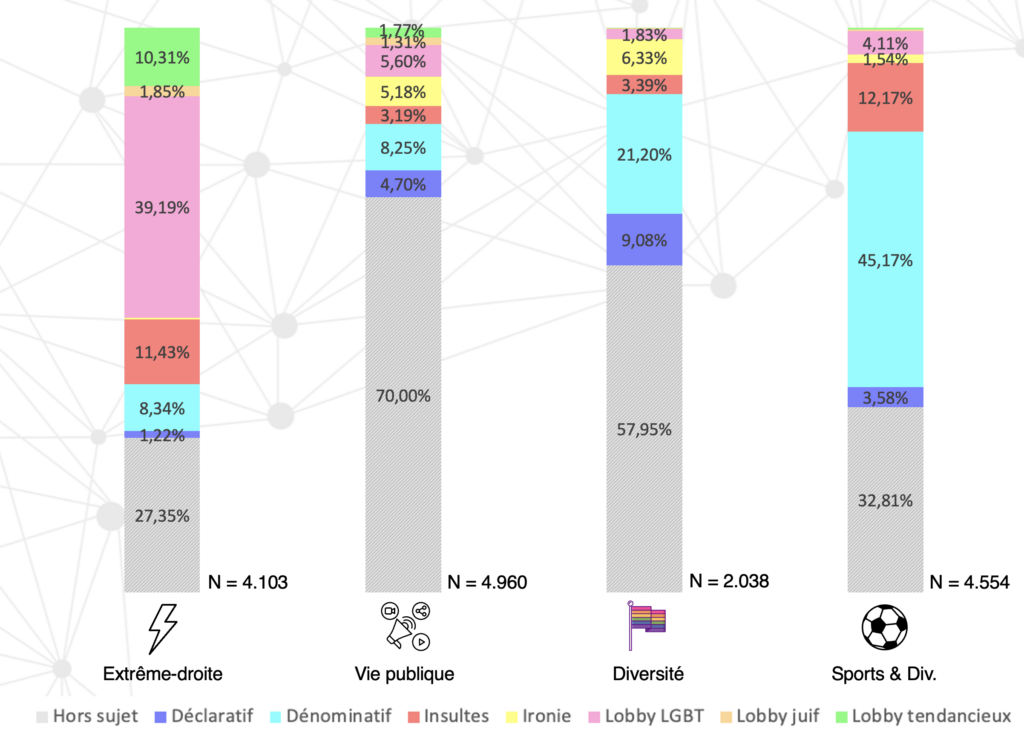
The most commonly used insults by these communities are as follows:
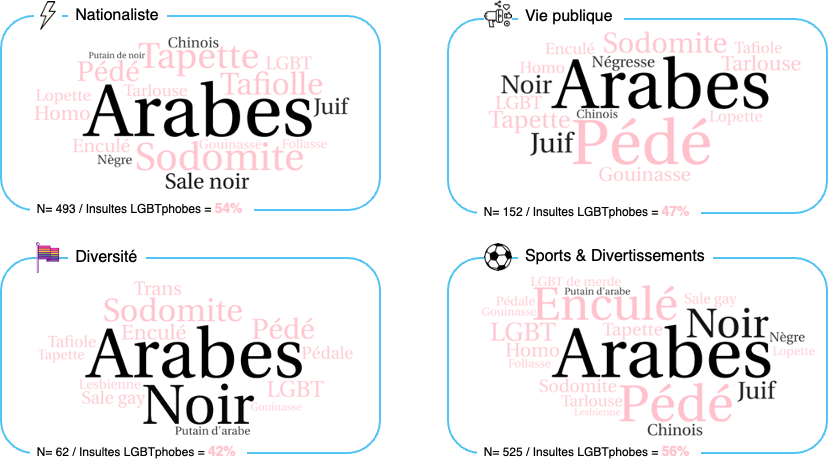
Lastly, in terms of insults, men and pseudonymous accounts are more likely to insult others, although only 4% of men engage in this behavior.
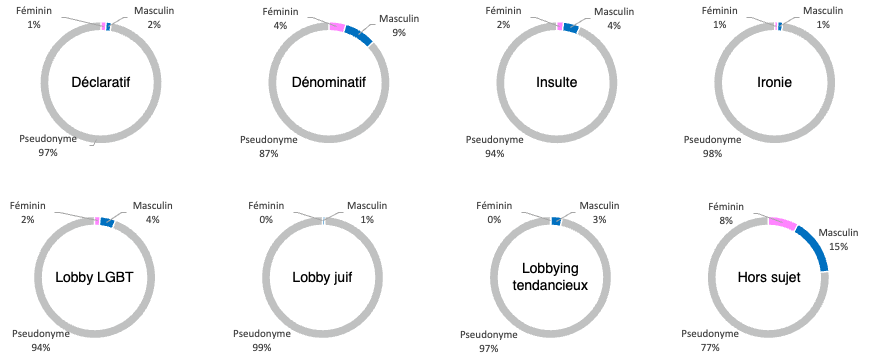
Methodology
We isolated several insults encompassing no fewer than 140,161 tweets over 13 months.

A filter was applied to remove irrelevant tweets, articles, and retweets, reducing the dataset to 10,861 unique tweets. All our analyses were conducted on this corpus (how the authors follow one another, tweet typology, and a qualitative analysis).
There are numerous challenges when dealing with hate speech. One of the most prominent issues is the semantic challenge due to the many different references:
•Fly swatter, mosquito swatter, or mouse swatter.
•Italian Democratic Party (PD).
•Responses to the account @Lobby_LGBT or Chinese restaurants.
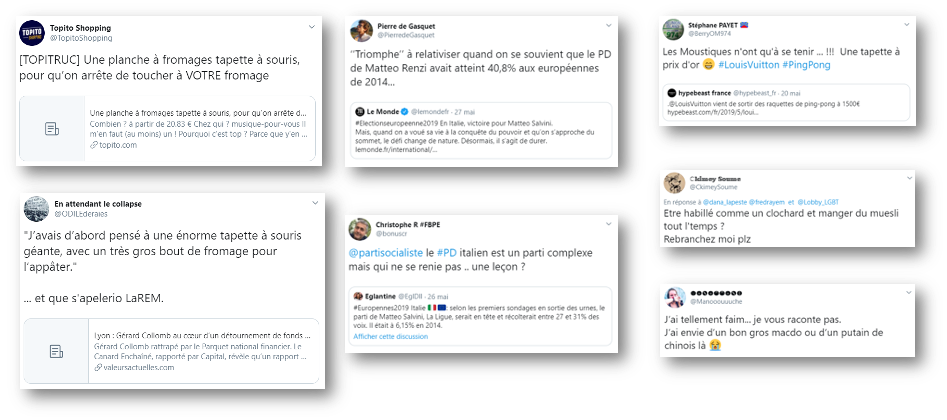
The contextualization problem: The offensive keyword is used in a non-violent context (e.g., H&M's “Bamboula” shirt).